- Type:
-
Seminar
- Time/Place:
-
Thursday, 13:00, Live/Video or Hybrid Seminar, Webex-Links are provided via the mor-seminar mailing list.
- Organizers:
-
ITM: apl.-Prof. Dr. Jörg Fehr
IAM (CE): Prof. Dr.-Ing. Felix Fritzen
IANS: Prof. Dr. Bernard Haasdonk
IANM (KIT): Prof. Dr. Benjamin Unger - Audience:
-
SimTech-PhD students, General interested audience in MOR, Surrogate Modelling, Control and Real-time Simulation from academia as well as industry.
- Goals:
-
Since 2009, this seminar represents a general platform for talks and exchange in the field of surrogate modelling, in particular Model Order Reduction (MOR) as well as novel data-based techniques in simulation science. Both methodological as well as application oriented presentations highlight the various aspects and the relevance of surrogate modelling in mathematics, technical mechanics, material science, control theory and other fields. We aim both at university members, as well as external persons from science and industry. The seminar is organized by four research groups and represents an activity of the SimTech Cluster of Excellence.
The presentations are announced some days in advance via the mor-seminar mailing list of the University of Stuttgart.In case of interest to join this mailing list, please contact the organizers or register at
https://listserv.uni-stuttgart.de/mailman/listinfo/mor-seminar
Presentations SS 2025
Title: A Trust-Region framework for Optimization using Hermite Kernel surrogate models
Abstract: In this talk, we present a trust-region optimization framework that employs Hermite kernel surrogate models. The method targets optimization problems with computationally demanding objective functions, for which direct optimization is often impractical due to expensive function evaluations. To address these challenges, we leverage a trust-region strategy, where the objective function is approximated by an efficient surrogate model within a local neighborhood of the current iterate. In particular, we construct the surrogate using Hermite kernel interpolation and define the trust-region based on bounds for the interpolation error. As mesh-free techniques, kernel-based methods are naturally suited for medium- to high-dimensional problems. Furthermore, the Hermite formulation incorporates gradient information, enabling precise gradient estimates that are crucial for many optimization algorithms. We prove that the proposed algorithm converges to a stationary point, and we demonstrate its effectiveness through numerical experiments.
Title: Impact Stress in a Rock Drilling Machine Piston
Abstract: TBD
This is an invited SimTech Colloquium:
Title: Nonlinear compressive reduced basis for the approximation of parameter dependant PDE's
Abstract : Approximating functions that depend on parameters can be greatly improved by first analyzing the
set of such functions as the parameters vary within a given domain. In the context of model reduction, this analysis
relies on various notions of N-width, such as Kolmogorov or Gelfand N-widths, which involve linear or nonlinear encoders and decoders. In simpler cases, this relates to classical techniques like Singular Value Decomposition (SVD) or Proper Orthogonal Decomposition (POD), while more advanced approaches may make use of neural networks for learning efficient representations.
In this talk, I will introduce these methods with a focus on their application to the approximation of solutions to parameter-dependent partial differential equations (PDEs), leading to what we refer to as nonlinear reduced
basis methods. I will then present practical numerical strategies for solving the resulting discrete systems, and share results on several challenging problems.
The results were obtained in collaboration with Hassan Ballout, Joshua Barnett, Albert Cohen, Charbel Farhat,
Christophe Prud'homme and Agustin Somacal.
Presentations WS 2024/2025
Title: Improving Eigensystem Realization Algorithm for Acoustics
Abstract: Modeling the complex dynamics of acoustical systems constructively can be challenging due to imprecise knowledge of material properties and domain geometry. Thus, measurements are often unavoidable in practice, and high-fidelity measurement data is abundantly available in the field. So far, reduced-order modeling methods are not widely used in acoustics. At the other end of the spectrum in mathematics, new methods are oftentimes only validated by means of synthetic data and toy models. Consequently, a noticeable gap between theory and practice remains. Our aims are twofold:
Firstly, clearing obstructions that arise when employing recent data-driven reduced order modeling methods in acoustical engineering practice.
Secondly, providing measurement data of real-world systems that can serve as benchmark problems for mathematicians developing new data-driven
methods.
In this talk, we showcase a selection of numerical issues that arise when applying the so-called Eigensystem Realization Algorithm by means of real examples. Amongst others, we consider head-related transfer functions that are used for auralization in virtual reality applications.
Title: Certified model order reduction of multi-physics systems
Abstract: In order to develop innovative products and understand complex processes, it is necessary to consider the various physical domains involved when modeling and simulating those systems. The port-Hamiltonian framework is particularly suitable for the simulation of multi-physics systems, which places the energy at the center of the modeling and thereby provides beneficial properties such as modularity and passivity. In the presentation, both the classical and the data-based modeling of port-Hamiltonian systems will be presented. To preserve the pH properties, it is necessary to reduce the systems in a structure-preserving manner. The possibilities and advantages of this structure-preserving model reduction will be demonstrated. Finally, a posteriori error estimation of the reduced systems is discussed.
Title: Efficient Surrogate Modelling for Hamiltonian Systems
Abstract: Efficient numerical simulation of large-scale dynamical systems, which may occur as Hamiltonian systems, poses significant
computational challenges. Traditional model order reduction (MOR) methods, such as the Proper Orthogonal Decomposition (POD), in general do not preserve the Hamiltonian structure. This may compromise energy conservation and stability in reduced-order models (ROMs). Structure-preserving approaches, such as symplectic MOR, address this issue by maintaining the Hamiltonian structure and therefore conservation and stability properties.
This presentation will outline two symplectic basis generation techniques, the randomized complex SVD (rcSVD) and dictionary-based cSVD (DB-cSVD), which improve the efficiency of basis generation for symplectic MOR of high-dimensional Hamiltonian systems and effectively address the challenges posed by slowly decaying Kolmogorov n-widths. Furthermore, an intrinsically symplectic kernel-based approximation scheme for learning Hamiltonian dynamics from time-series data will be discussed. This method employs greedy kernel selection to achieve efficient and accurate flow map approximation by interpolating suitable generalized interpolation conditions.
Title: Towards real-time exploration of grain boundary effects on diffusion in solid electrolytes
Major progress in battery technology is key to decarbonization. In All-Solid State Lithium Ion Batteries (ASSLIBs), the liquid electrolyte is replaced by a polycrystalline solid-state electrolyte, promising increased capacity, safety, and performance.
The diffusion behavior of Li ions in the solid-state electrolyte is crucial for battery performance. Atomistic studies indicate that grain boundary (GB) effects and grain size significantly impact diffusivity. Advances in active learning methods have enabled large-scale, high-accuracy simulations using ab initio-based machine-learning interatomic potentials. However, these approaches are computationally demanding, leaving a gap between atomistic and application scales. Larger-scale simulations, however, often neglect polycrystalline structure details or assume isotropy.
Our approach considers the fully resolved crystalline structure with a parametrization aligned with the atomistic perspective to describe diffusion along and across GBs. This is embedded into a finite element simulation using a novel collapsed interface element. Results are governed by different and potentially anisotropic diffusion coefficients in bulk and GB domains. An effective mesoscale model is derived using homogenization based on a periodic reference volume element. The hybrid bulk-interface diffusion model allows for a quantitative study of GB contributions to overall diffusivity.
The affine structure of the problem is exploited to derive a reduced-order model. A few full simulations enable accurate real-time estimates for effective diffusion coefficients across the parameter space. The reduced-order model allows in-depth analysis of material and structural features governing diffusion behavior, facilitating in silico exploration of microstructures and their implications on larger scales for designing improved materials and batteries.
Title:
Nonlinear Model Reduction using Kernel Methods and Autoencoders
Abstract:
This talk explores data-driven techniques to identify low-dimensional modeling variables for nonlinear dynamical systems. The common wisdom is that such variables should provide an embedding that allows to accurately reconstruct the state variables near trajectories of interest, or near an underlying invariant manifold. Paradoxically, methods such as proper orthogonal decomposition and autoencoders trained to reconstruct high-dimensional states can result in models that utterly fail to make accurate forecasts for systems with fast dynamics or nonnormal sensitivity mechanisms. We aim to explain why these methods fail, and to introduce techniques that overcome these limitations. To do this, our new methods leverage data collected from simulations of the nonlinear system together with adjoint-based linearized sensitivity analysis to identify low-dimensional variables suitable for making forecasts. We discuss linear and nonlinear dimensionality reduction using kernel methods and autoencoders, and we apply these techniques to problems in fluid dynamics.
Title: Projection-based model order reduction for large-scale parametric eigenproblems
We deal with efficient and certified numerical approximation of
parametric Hermitian eigenproblems related to the smallest eigenvalue
and its associated eigenspace.
For this aim, we rely on projection-based model order reduction (MOR),
i.e. we approximate the large-scale problem by projecting it onto a
suitable subspace, reducing it to a much smaller dimension.
Such a subspace is constructed by means of weak-greedy type strategies
applied either on a continuum or discrete parameter domain. We
introduce a novel error estimate for the eigenspace associated with the
smallest eigenvalue. It turns out that the difference between the
second smallest and the smallest eigenvalues, the so-called spectral
gap, is crucial for the reliability of the error estimate.
Therefore, we propose new efficiently computable upper and lower bounds
for the spectral gap,
which enable the assembly of a subspace for the certified MOR
approximation of the spectral gap. Based on that, a second subspace is
generated for a certified MOR approximation of the eigenspace
associated with the smallest eigenvalue. Our method is well-suited to
tackle the cases where the smallest eigenvalue is not simple.
This work is motivated by a particular application: the repeated
evaluation of the ground state of parametric quantum spin system (QSS)
models. In this framework, the ground state corresponds to the
eigenspace associated with the smallest eigenvalues of the QSS
Hamiltonian.
Presentations SS 2024
Title: "Robust Sparse Model Discovery"
Rapid advances in material science and additive manufacturing are enabling a new class of adaptive autonomous systems, such as morphing wing drones and soft robotic swimmers. These systems can adapt their shape to uncertain and rapidly changing flow conditions and are likely to provide superior performance compared to conventional rigid system, as demonstrated by the incredible performance of birds and insects that exploit the flexibility of their wings to perform agile and efficient flight maneuvers. Several challenges remain in understanding how these adaptive wings are designed and optimized to achieve system-level performance gains, and how efficient control of the nonlinear fluid-structure dynamics is achieved. In particular, the intertwined processes of optimizing the wing design and learning a control policy present a great challenge.
In this talk, I will discuss ideas around co-design optimization and data-driven modeling and control, with applications in adaptive structures and morphing wings. I will introduce the sparse identification of nonlinear dynamics (SINDy) method, and recent work on extending the SINDy method using ensemble learning. I will show that the ensemble statistics from Ensemble-SINDy can be used for active learning, improved model predictive control, and sample efficient reinforcement learning.
Webex link: https://unistuttgart.webex.com/unistuttgart-en/j.php?MTID=mace1c7baf033601325c465aa750776a7
Title: Learning Parameter-dependent Hamiltonian Systems with Structure-preserving Neural Networks
Abstract: When solving Hamiltonian systems numerically, it is essential to preserve the symplectic structure of the flow map. Symplectic numerical integrators help with long-term energy conservation and stability. Different approaches to symplectic structure-preserving neural networks have been developed. However, no symplectic neural networks have been developed for parameter-dependent Hamiltonian systems, so far.
We propose a neural network architecture that can learn a parameter-dependent flow map, while preserving the symplectic structure. We compare these neural networks against physics-unaware multilayer perceptrons, for which integrating a parameter dependency can be easily achieved by adding an additional input neuron. The structure-preserving neural networks are able to extrapolate to areas of the phase space without training data, while the physics-unaware multilayer perceptrons are not. Furthermore, they can learn the underlying dynamics with very sparse data compared to the multilayer perceptrons. The structure-preserving neural networks achieve all of this with the same prediction speed as multilayer perceptrons.
Title: Machine learning chemical reaction models using physical background knowledge
Abstract:
Many important processes like technical reactors, catalysis, combustion, atmospheric chemistry, system biology or the synthesis of battery materials are controlled by complex reaction mechanisms. We will demonstrate how embedding a priori knowledge into neural networks allows efficient machine learning of kinetic models for these systems. One example is our recently introduced Global Reaction Neural Network that implements stoichiometric and thermodynamic consistency in the neural network structure and learns a latent representation of global reaction rates [1]. Another example are chemical reaction neural networks with a dedicated atom balancer layer [2]. We will discuss the application of these machine learned models as surrogates to speed up system simulations. If a reactor model is included in the backpropagation training loop (Neural ODE), kinetic models can be directly learned from reactor data. We will demonstrate that this approach accurately recovers kinetic models from big data sets, even in the presence of substantial noise. This will enable the application in production environments, where due to digitization and the availability of cheap sensors increasing amounts of frequently low quality data are becoming available. Finally, we will present recent work in collaboration with the Haasdonk group in Stuttgart where we replace Neural Networks by deep kernel methods, yielding models with improved error estimates [3]. Prospects and challenges of this recent development will be discussed.
The talk will also provide an overview Umicore’s most important business areas and how simulation is applied to accelerate product and process development.
Presentations WS 2023/2024
Title: Passivity preserving model reduction via spectral factorization for descriptor systems
Abstract: The objective of this talk is passivity-preserving model reduction for descriptor systems. We show how the recently developed method "Passivity preserving model reduction via spectral factorization" [1] for standard systems can be extended to descriptor systems. We develop the necessary theory and compare the numerical results to other available methods. It turns out that in our numerical examples the spectral factorization method outperforms the passivity-preserving method "positive-real balanced truncation" [2] in the H2-norm. The spectral factorization method produces reduced-order models close to unstructured H2-optimal reduced-order models. Our numerical experiments indicate that it might be favorable to apply model order reduction directly on the original full-order model without reducing its system index. The talk is closely related to generalized variants of the Kalman-Yakubovich-Popov (KYP) inequality for descriptor systems.
[1] T. BREITEN AND B. UNGER. Passivity preserving model reduction via spectral factorization. Automatica, 142:110368, 2022.
[2] T. REIS AND T. STYKEL. Positive real and bounded real balancing for model reduction of descriptor systems. International Journal of Control, 83(1):74–88, 2010.
Title: Data-driven-based History-Dependent Surrogate Models in the context of stochastic multi-scale simulations for elasto-plastic composites
Abstract:
When developing stochastic models or performing uncertainty quantification in the context of multiscale models, considering direct numerical simulations at the different scales is unreachable because of the overwhelming computational cost. Surrogate models of the micro-scale boundary value problems (BVP), typically Stochastic Volume Elements (SVE), are then developed and can be constructed or trained using off-line simulations. In such a data-driven approach, different kinds of surrogate models exist including in the context of non-linear behaviours, but difficulties arise when irreversible or history-dependent responses have to be accounted for as in the context of elasto-plastic composites. In this paper we investigate three kinds of surrogate models that can handle elasto-plasticity.
Once trained using a synthetic database, neural-networks (NNWs) can substitute the micro-scale BVPresolution while reducing the computation time by more than 5 orders of magnitude. In the context of reversible behaviours or proportional loading, feed-forward NNWs can predict a homogenised response, possibly for different parametrised micro-structures. In order to introduce the history dependency, recurrent neural networks (RNNs) were shown to be efficient and accurate in approximating the history-dependent homogenised stress-strain relationships. The limitations of NNWs are mainly two-fold. On the one hand they are unable to extrapolate responses (they can only interpolate), and on the other hand they require a large synthetic database to be trained. A physics informed alternative is the deep material network (DMN) approach which consists in a network of mechanistic building blocks. During the training process, the DMN “learns” the weight ratio and interactions of the building blocks. Once trained, the DMN is able to predict nonlinear responses, including for unseen material responses and loading conditions, in a thermodynamically consistent way, although they are less computationally efficient than the NNWs in their online stage.
A last approach is to identify the parameters of a semi-analytical mean-field-homogenization (MFH) model from the resolutions of different micro-scale BVP or SVEs: a set of MFH parameters is associated to each SVE. Since the surrogate is purely micro-mechanistic, it can handle damage-enhanced elasto-plasticity including strain-softening by considering objective quantities such as the critical energy release rate.
The different surrogates are applied in two different contexts: On the one hand the Bayesian inference of multi-scale model parameters and on the other hand, the stochastic multi-scale simulation of composite coupons.
Title: “Reduced basis method for quantum spin systems”
Abstract:
In this talk, we present a reduced basis method for quantum spin problems. Within the reduced basis methods approach, an effective low-dimensional subspace of a quantum many-body Hilbert space is constructed in order to investigate, e.g., the ground-state phase diagram. The basis of this subspace is built from solutions of snapshots, i.e., ground states corresponding to particular and well-chosen parameter values. Here, we show how a greedy strategy to assemble the reduced basis and thus to select the parameter points can be implemented based on matrix-product-states (MPS) calculations using DMRG-optimization. Once the reduced basis has been obtained, observables required for the computation of phase diagrams can be computed with a computational complexity independent of the underlying Hilbert space for any parameter value. We illustrate the efficiency and accuracy of this approach for different one-dimensional quantum spin-1 models.
Title: Numerical Surrogate Models for the Optimization of Automotive Crash Structures
Abstract: A challenge in the design and optimisation of vehicle structures is the high computational costs required for crash analysis. A methodological approach to simplifying Finite Element (FE) vehicle models and crash barriers is presented in this talk. The methodology uses global deformation characteristics of structures which are obtained from the global crash model. For the simplification of the vehicle crash model structural regions which are sustaining only elastic deformations during the crash are replaced by kinematic numerical representations which describe both stiffness and load paths at the interface of the substituted structures. Verification studies of the simplified vehicle model show a very good agreement of the global and local structural response during the frontal crash. Further simplifications were applied to deformable barriers by replacing its detailed crushing behaviour by kinematic descriptions. Through the combined use of both simplified numerical representations,
the computational cost of an Euro NCAP offset crash analysis can be reduced by around 92%. With the obtained time reduction structural optimisation studies of the remaining structure can be conducted efficiently for the identification of weight reduction potentials.
Title: "Optimal Feedback Law Recovery by Gradient-Augmented Sparse Polynomial Regression“
Abstract:
In this talk, our objective is to approximate the solution of Hamilton-Jacobi-Bellman (HJB) equations associated with a class of optimal control problems using machine learning techniques. As known in optimal control theory, the solution to HJB equations provides the optimal values for associated optimal control problems with different initial data, and its gradient is used to formulate the optimal feedback control policy. Due to the so-called "curse of dimensionality," directly solving HJB equations becomes numerically intractable for high-dimensional optimal control problems.
We present a data-driven approach for approximating the solution of HJB equations for general nonlinear problems and computing the related optimal feedback controls. This approach leverages the control-theoretical connection between HJB and first-order optimality conditions through Pontryagin's Maximum Principle. It is based on the following key elements: generating a random dataset consisting of different state-value pairs, approximating the value function sparsely using orthogonal polynomials, and recovering sparsity through a (weighted LASSO) $\ell_1$-minimization decoder. We also provide numerical experiments that demonstrate how enriching the dataset with gradient information reduces the required number of training samples, and how sparse polynomial regression consistently results in a feedback law with lower complexity.
Presentations SS 2023
Title: Error-controlled sparse collocation of reduced order models and applications in uncertainty quantification for vibroacoustics
Abstract: This talk is concerned with efficient surrogate modeling of parametric vibroacoustic problems in the frequency domain. We consider both frequency and random parameters, where in particular random influences quickly lead to high-dimensional problems, which typically result in a high computational effort. Recently, the interpolation of local reduced order models (ROMs) is receiving increasing attention, in part because sparse collocation allows to tackle high-dimensional problems. In this case, in addition to finite element discretization errors, at least two sources of numerical error arise, which need to be controlled. For a fixed random parameter, we consider moment matching ROMs, where an adjoint problem is used to cheaply and accurately estimate the error. This in turn allows for an adaptive selection of the frequency snapshot locations and the ROM moment-matching order. We then collocate these local ROMs on a dimension-adaptive or locally refined sparse grid, where another adjoint problem is used for error control and adaptivity. Dimension-adaptive sparse grids can exploit a possible parameter anisotropy, whereas local refinement is needed for systems with low damping, where the response surfaces exhibit strong variations. Overall, we obtain a numerical method, which is applicable in high dimensions and which features a high degree of flexibility. Given a prescribed error budget, the different error contributions can be balanced by adjusting the local ROM sizes and collocation grid. We will demonstrate the accuracy of the estimators and the capabilities of the method with several examples with a focus on vibroacoustics and the computation of statistical moments and global sensitivities.
Title: Low dimensional surrogate modeling for port-Hamiltonian systems
Title: Numerical model reduction with error control for multiscale modeling of porous media
Abstract: Computational homogenization can be used in order to model the effective mechanical behavior of fluid saturated porous media with heterogeneous properties. A standard approach is the "finite element squared" (FE2) procedure, where a new boundary value problem for the coupled porous media problem is defined on a Representative Volume Element (RVE). The effective macroscopic response is obtained from solving the RVE problem. The FE2 strategy can be computationally expensive and it is therefore of interest to reduce the cost of solving the individual RVE problems by introducing a reduced basis. A well known method for identifying a reduced basis is Proper Orthogonal Decomposition (POD). Typically POD basis reduction can be split into an "offline" stage and an "online" stage. During the offline stage a set of training computations are performed in order to collect snapshots of the system. These snapshots are then used to construct a POD basis. In the online stage the simulation is accelerated by using the reduced basis. Naturally the accuracy of the online simulation depends on how well the reduced basis can capture also the new conditions. In this presentation we will present a POD-reduced model for solving the RVE problem, together with an a posteriori error estimator. We will also present some initial work towards an adaptive method, where the reduced basis is updated during the online stage to better capture new, previously unseen, loading.
Presentations WS 2022/2023
Program Flyer
Program Flyer
the public Argyris Lecture gives leading personalities in the field of simulation science the opportunity to present their research to a broad audience. We are proud that for the year 2022 Prof. Serkan Gugercin received the Argyris Vising Professorship. Prof. Serkan Gugercin stands out for his excellent achievements and research results in the field of Model Order Reduction (MOR) and surrogate modelling. We would like to use the occasion of his Argyris Lecture on 07. December 2022 to introduce a broad audience to the latest trends and methods in the diverse world of model order reduction. We therefore warmly invite you to the “MOR-Day” on 07. December 2022. The program starts at 13:30 h in V7.31 with four talks from different MOR groups from the University of Stuttgart:
- Patrick Buchfink, 13:30 – 14:00: "Model order reduction on manifolds"
- Jonas Kneifl, 14:00 – 14:30, "Real-time Human Response Prediction in Integrated Safety Situations Using Model Reduction and Machine Learning"
- Jonas Nicodemus, 14:30 – 15:00: Towards multi-objective optimization for reduced port-Hamiltonian systems
- Felix Fritzen, 15:00 – 15:30, Reduced order modelling of linear and nonlinear thermo-mechanical materials
After a short break with a room change, we will meet at 16:00 h in V7.01 for the exciting Argyris Lecture by Serkan Gugercin.
- Prof. Serkan Gugercin, 16:00 – 17:30, V7.01: "Modeling dynamical systems from data: A systems-theoretic perspective"
A reception in the foyer of the PWR5a building will round off the day with enjoyable conversation, drinks and food. With a drink in hand, the poster exhibition of further MOR groups can also be explored there.
Title: Learning dynamics of nonlinear systems via structure-preserving operator inference.
In this talk, we will discuss several extensions of a data-driven structure-preserving
model reduction framework via operator inference. We will illustrate how that framework
learns reduced-order models non-intrusively, and how additional knowledge—which is often
present about fluid dynamical and other mechanical systems—can be embedded as constraints
for the resulting optimization problem. Three aspects
will be discussed: First, we will present variable transformations to turn highly nonlinear
models into quadratic form, which can then be straightforwardly parametrized and learned.
We will illustrate the results on a 2d rocket combustion application. Second, for
energy-preserving systems, we will embed Hamiltonian structure into the model learning
framework, which guarantees that the learned models are long-term stable and energy-conserving.
We will illustrate those results on a wave equation and the nonlinear Schroedinger equation.
Lastly, we present recent results for stability-domain estimates for quadratic systems,
and how those can be used to regularize the learning problem to guarantee stable models,
illustrated on the quadratic Burgers’ equation.
Title: The influence of the Hamiltonian in structure-preserving model reduction
Computing reduced-order models for large-scale systems that arise, e.g., from a spatial semi-discretization of a partial differential equation has become one of the standard techniques for efficient control and simulation of complex dynamical processes. In the case that the original model has a particular structure, one often aims at a structured
surrogate model. This is particularly true for the class of port-Hamiltonian systems for which several structure-preserving techniques have been developed recently. However, basically all existing methods ensure structure-preservation by utilizing the Hamiltonian matrix within the projection step. Proceeding this way obviously reduces the degree
of freedom for a Petrov-Galerkin projection as one of the matrices is pre-determined by the Hamiltonian. Moreover, the approximation quality implicitly depends on the specifically chosen realization of the port-Hamiltonian system, raising the question of existence of an optimal Hamiltonian with regard to model order reduction. This talk discusses particular choices for the Hamiltonian with regard to structure preserving balancing and interpolatory based model reduction methods.
This is joint work with Riccardo Morandin, Philipp Schulze and Benjamin Unger.
Title: Surrogate Models from Simulation Data in Structural Dynamics
Abstract:
Surrogate modeling of structural dynamics systems such as finite element simulation models faces significant challenges.
Some of the reasons are (i) the sheer dimensionality of such systems, (ii) the inaccessible code of commercial software, and (iii) the computationally intensive data generation.
Data-based non-intrusive model order reduction has emerged as a potent solution to the task of creating efficient yet accurate surrogate models for these kind of systems, addressing two
main problems.
One is the identification of coordinates that are simultaneously low-dimensional to ensure computational efficiency, yet still adequately describe the system and its dynamics.
The other is the approximation of the (parameter-dependent) system behavior in the identified reduced coordinates.
In this talk we will present different methods for both steps and discuss their advantages and disadvantages in different situations including dynamic simulations of human body models and
simplified crash scenarios.
Title: Physics-aware machine learning in the small-data regime: from random walkers to random media
Presentations SS 2022
Program Flyer
Program Flyer
Title: Symplectic Model Reduction of Hamiltonian Systems on Nonlinear Manifolds
Title: Brain Networks, Gas Networks and Model Reduction
Abstract:
Dynamic network behavior is a research object in various sciences and not least in applied
mathematics: Network dynamics are studied in biological systems, such as the brain, in technical systems such as gas networks, or abstractly in neural networks.
Realistic models of such large networks not only comprise a high dimensionality either due to a large number of nodes, or mathematical conditions such as hyperbolicity, but also due to
complexities like (non-smooth) nonlinearities. Now, simulating network control or observations requires repeatedly solving a large-scale nonlinear dynamic system for many input or parameter configurations. Especially in time-constrained settings like short-term forecasts, the question if these simulations can be accelerated, arises. Data-driven system-theoretic model reduction is a remedy for this problem: A dynamic network model can be formulated as a (nonlinear) input-output system and based on the system-theoretic properties, which are approximated from simuations, a reduced order model can be computed. This approach is not unlike unsupervised learning, but instead of learning a surrogate model, rather
its system-theoretic properties, and thereby its redundant and irrelevant components in terms of measurable input-output behavior, are obtained. A generic projection-based model order reduction framework for this approach is presented, together with a discussion of particular challenges and opportunities of neuronal brain networks and gas transport networks, as well as numerical examples alongside ideas for comparing reduced order models heuristically.
Title: Compatible Port-Hamiltonian Discretization and Model Reduction of a Nonlinear Flow Problem
Abstract:
Structure-preserving approximation is still an active research area. By preserving or mimicking relevant geometric structures such as, e.g., conservation laws or symplecticities, unphysical solution behavior and numerical instabilities can be avoided in many cases. The model problem considered in this contribution describes nonlinear flows on networks. It covers a hierarchy of models used to describe gas network systems, including particularly the barotropic Euler equations.
Our discretization and model reduction approach is analyzed using energy-based modeling concepts, such as the port-Hamiltonian formalism and the so-called partial Legendre-transformation. The latter offers an elegant approach for the systematic analysis of certain variable transformations, which widens the range of formulations, for which structure-preserving Galerkin-type approximations can be derived under a few compatibility conditions, using variational arguments only.
A particular focus of the talk also lies on the realization of the snapshot-based model order- and complexity-reduction. While beneficial for the robustness and performance of the reduced models, the compatibility conditions pose a challenge in the training phase. Appropriate adaptions of the conventional model reduction methods will be presented.
Title: Microstructure property prediction through neural networks using POD, geometric and convolutional features
Presentations WS 2021/2022
Program Flyer
Program Flyer
Webex link: https://unistuttgart.webex.com/unistuttgart/j.php?MTID=m965727048b34537876820118921cda05
Title: Data-driven complexity reduction of dynamical systems from frequency and time-domain measurements
Authors: Ion Victor Gosea, MPI Magdeburg, Germany (joint work with Serkan Gugercin and Christopher Beattie, Virginia Tech, Blacksburg, Virginia, USA)
Abstract:
In many engineering applications, the underlying dynamics of the process to be studied may be inaccessible to direct modeling, or it may be only partially known. However, with the ever-increasing prevalence of available data from various experiments, it is of interest to incorporate measurements into the modeling and complexity reduction process. Data corresponding to the underlying dynamical system (usually complex or of large scale) are available in various formats. For example, in the form of the frequency response (transfer function evaluations) or of input-output values measured in the time domain. In such cases, one could construct a simplified empirical
model of lower dimension that fits the measured data, and hence accurately approximates the original dynamical system. This reduced-order system may then be used as a surrogate to predict behavior, derive control strategies, whenever the desired performance is sensitive to the model order.
The main motivation of the proposed method comes from the fact that we can use system response data (in compressed format) that has been either measured or computed, without the need of accessing any prescribed realization of the original model. Data are represented by sampled values of the transfer function corresponding to the original model (in the frequency domain), or of evaluations of the impulse response (in the time domain). It is to be noted that we do not require at any time explicit access to the state variable of the system,
sampled at different time instances. The quantities that we need in the proposed data-driven approach are system invariants, i.e., they are not altered by coordinate transformations, and their dimensions do not scale with the number of variables (model order) needed to represent the original dynamics.
We discuss parallels that our approach bears with balanced truncation (a common model reduction method), the possibility of deriving error bounds to quantify the distance to the truth model (if known), as well as connections to the Loewner framework, a popular data-driven method. We illustrate our approach numerically for various models and data sets.
Webex link https://unistuttgart.webex.com/unistuttgart/j.php?MTID=mea13a9d845111c136e37d46dc88f1566
Title: Modelpredictive Wind Turbine Control – Modelling, Implementation, Field-Testing and Perspectives
Abstract: Due to its high energy density, wind turbines represent one of the main sources of tomorrow's energy
supply among the renewable energy sources. Because of the fluctuating wind conditions, the control
strategy is of central relevance. While the maximum yield is one of the central control objectives due
to the current legal situation, further control objectives such as economic efficiency, structural
integrity and grid services will be added due to future requirements. One possibility to optimally design
these control objectives is the model predictive control. In the lecture, the work of several research
projects between the Institute of Automatic Control at RWTH Aachen University and the plant
developer W2E will be presented, from the first simulative tests to the field test. The main focus of the
presentation will be on the aeroelastic modelling, the systematic implementation and the
experimental setup up to the field test. Especially for the field test on industrial hardware, quickly
computable surrogate models/process models are necessary. These models are obtained with dataand
physics-based approaches and a model order reduction of complex simulation models. Finally,
future perspectives will be shown, such as how structural health monitoring can be integrated into the
model predictive control.
Webex-link: https://unistuttgart.webex.com/unistuttgart/j.php?MTID=m62cbb7d6e1b4f64b59d4c64589935bd0
Teaser-Video: https://www.mib.uni-stuttgart.de/dae/video/mor_seminar_teaser.m4v
Title: "A Recurrent Neural Network-based Surrogate Model for History-Dependent Multi-scale Simulations"
Abstract: Homogenization-based multi-scale analyses are widely used to account for the effect of material heterogeneity at a structural material point. Among the existing different homogenization methods, computational homogenization solves the meso-scale heterogeneous problems using a full field discretization of the micro-structure. When embedded in a multi-scale analyses, computational homogenization results in the so-called FE2 method, which is an accurate methodology but which yields prohibitive computational time. A more efficient approach is to conduct pre-off-line finite element simulations on the meso-scale problem in order to build a surrogate model by means of constructing mapping functions. Once this so-called training step is completed, the surrogate model can be used as the constitutive law of a single-scale simulation, leading to highly efficient simulations. Artificial neural networks (NNWs) offer the possibility to build such a mapping. However, one difficulty arises for history-dependent material behaviours, such as elasto-plasticity, since state variables are needed to account for the loading history. This difficulty can be solved by considering a Recurrent Neural Network (RNN), which uses sequential information. In [1] a RNN was designed using a Gated Recurrent Unit (GRU). In order to achieve accuracy under multi-dimensional non-proportional loading conditions, the sequential training data were obtained from finite element simulations on an elastoplastic composite RVE subjected to random loading paths. The RNN predictions were found to be in agreement with the finite elements simulations. This project has received funding from the European Union’s Horizon 2020 research and innovation programme under grant agreement No. 862015 for the project “Multi-scale Optimisation for Additive Manufacturing of fatigue resistant shock-absorbing MetaMaterials (MOAMMM)” of the H2020-EU.1.2.1. - FET Open Programme.
[1] Wu L, Nguyen V-D, Kilingar NG, Noels L. A recurrent neural network-accelerated multi-scale model for elasto-plastic heterogeneous materials subjected to random cyclic and non-proportional loading paths. Computer Methods in Applied Mechanics and Engineering 360, 2020, p. 113234
Webex-link: https://unistuttgart.webex.com/unistuttgart/j.php?MTID=mf10552ebc5ca1f9f0a07c7d039816d99
Title: Structure-preserving dynamical reduced basis methods for Hamiltonian systems
Abstract: In this talk, we will consider reduced basis methods (RBM) for the model order reduction of parametric Hamiltonian dynamical systems describing nondissipative phenomena. The development of RBM for Hamiltonian systems is challenged by two main factors: (i) failing to preserve the geometric structure encoding the physical properties of the dynamics, such as invariants of motion or symmetries, might lead to instabilities and unphysical behaviors of the resulting approximate solutions; (ii) the local low-rank nature of transport-dominated and nondissipative phenomena demands large reduced spaces to achieve sufficiently accurate approximations. We will discuss how to address these aspects via a structure-preserving nonlinear reduced basis approach based on dynamical low-rank approximation. The gist of the proposed method is to evolve low-dimensional surrogate models on a phase space that adapts in time while being endowed with the geometric structure of the full model. If time permits, we will also discuss a rank-adaptive extension of the proposed method where the dimension of the reduced space can change during the time evolution.
Webex-link: https://unistuttgart.webex.com/unistuttgart/j.php?MTID=mbbc62b745ee3af74a4b7f6565676526c
Title: On modeling and discretization from a port-Hamiltonian perspective
Abstract: The port-Hamiltonian formalism, founded 30 years ago, is powerful for control-oriented modeling, numerical approximation and order reduction of interconnected, multi-physical systems. Port-Hamiltonian models are at the core of energy-based control designs like Control by Interconnection or IDA-PBC. In the last decade, preserving the port-Hamiltonian structure in spatial and temporal discretization and reduction has been an active field of research with results in many directions. The goal of this talk is to give an introduction to geometric discretization, which emphasizes the underlying physics. In the first part, systems of conservation laws, their discrete modeling on dual topological objects and their geometric approximation are addressed. The second part is devoted to symplectic time integration and its use for discretization of control systems and control.
Presentations SS 2021
Program Flyer
Program Flyer
Webex: https://unistuttgart.webex.com/unistuttgart/j.php?MTID=mbf09de308226a023acc0c7c19ede65d7
"Model reduction for transport phenomena via state-dependent projections"
The standard task of projection-based model-order reduction (MOR) consists of finding a suitable low-dimensional subspace such that the solution of the problem at hand approximately evolves within this subspace. Hereby, the best subspace of a given dimension and the corresponding worst-case approximation error are quantified by the Kolmogorov n-widths. If the n-widths have a slow decay, which is typical for transport phenomena, then a good approximation with a low-dimensional subspace cannot be expected. To overcome this issue, we present a novel model reduction framework that allows the low-dimensional subspace to evolve along with the solution of the problem. Our MOR framework is inspired by the moving finite element method, yielding a nonlinear projection approach. The resulting reduced model is designed to minimize the residual, which is also the basis for an a posteriori error bound. In this talk, we discuss numerical aspects of our method, focusing on an efficient offline-online decomposition. The findings are illustrated with a wildfire application.
Webex: https://unistuttgart.webex.com/unistuttgart/j.php?MTID=m93e4574d05fb25e51f5a6ae906a8eb65
"Circumventing the limitations of projection-based model order reduction with random sketching"
This talk presents novel randomized projection-based model order reduction (MOR) methods for solving large-scale parameter-dependent linear systems. We use randomized linear algebra to address few central challenges of projection-based MOR such as: i) a high offline computational cost and the need to adapt the algorithms to modern computational architectures; ii) the requirement of approximability of the solution manifold in a low-dimensional space, that may not hold for complex problems; iii) the need of effective certification of the reduced order model, and the stability issues related to the high condition number of the operator. Our methods rely on random sketching that consists in random embedding a set of high-dimensional vectors, defining the problem of interest, into a low-dimensional space by almost preserving the pairwise inner products in the set, and then building the reduced order model in this low-dimensional space with a negligible computational cost. Random sketching algorithms are universal and can be adapted to practically any computational architecture by considering appropriate embedding matrices. We present new efficient, randomized versions of Petrov-Galerkin and minimal residual projection methods for finding an approximate solution in a low-dimensional space. Then it is shown how to efficiently
generate a basis for this space with the associated greedy algorithm or randomized Proper Orthogonal Decomposition. Furthermore, we incorporate the ideas from compressed sensing and random sketching to develop a dictionary-based
approximation method for problems with solution manifolds that can not be well approximated by a single low-dimensional space. This method proceeds with approximation of the solution by a projection onto a subspace spanned by several
vectors selected online from a set of candidate basis vectors, called dictionary. In its turn, such projection is obtained by an approximate solution of a large parametric sparse least-squares problem. In this context, random sketching plays the key role for the efficient (approximate) solution of this problem. Finally, we present strategies to construct a parameter-dependent preconditioner for the solution of ill-conditioned parametric systems and an effective error estimation/certification without the need to estimate expensive stability constants. The preconditioners are constructed by an interpolation of the inverse operator based on online minimization of an error indicator. We present several error indicators depending on the objective such as improving the quality of Petrov-Galerkin projection or residual-based error estimation. The associated heavy computations in both offline and online stages are circumvented by extending the methodology from random embeddings of vectors to random embeddings of operators.
Webex: https://unistuttgart.webex.com/unistuttgart/j.php?MTID=m7891d2bd099f2316ae1982f4c8ee55f2
"Model Order Reduction for Lithium-Ion Batteries using Reduced Basis Methods"
Webex: https://unistuttgart.webex.com/unistuttgart/j.php?MTID=md2a77215c62970e2625cf1aed7320621
"Model Order Reduction Strategies in Structural Mechanics: A Selection of Eigenvalue-Analysis-Based and Data-Driven Approaches"
In order to make reliable decisions, engineers must rigorously investigate large numerical complex finite element models considering nonlinear material behavior. However, such large-scale dynamic investigations are time-consuming and require storing vast amounts of data. I will present an ongoing development of model order reduction techniques applicable to linear and nonlinear structural mechanics. The proposed strategies entail applications and extensions of the proper orthogonal decomposition, the proper generalized decomposition, substructure techniques, and methods that include artificial neural networks.
Presentations WS 2019/2020
Program Flyer
Tensor approximation meets model order reduction
Certified Reduced-Order Modeling for Multiobjective, Nonsmooth and Stochastic Optimizatio
Reduced Order Modeling via Computer Vision in Solid Mechanics
A priori reduced order modelling in fluid-structure interaction.
This presentation focuses on Reduced Order Modelling (ROM) techniques
adapted for Fluid-Structure Interaction (FSI) problems. The prediction
of the coupled dynamic behaviour of elastic structures in contact with
fluids (liquid or gas) is still a challenging industrial and research
topic area. Examples of application can be the design of space launchers
with liquid propellants [1] or the harvesting of electrical energy via
flutter-induced vibrations [2]. The challenges arising from such
problems are: (a) to model and predict accurately the fluid-structure
system state for a given range of time/frequency and model parameters,
(b) allowing sensitivity analyses of the quantities of interest at the
system level under varying conditions which typically requires a large
number of numerical simulations, and (c) to incorporate real-time
feedback to allow optimal control of the system state (e.g. control of
trajectory or minimize the exposure to fatigue). Reduced order
techniques play a crucial role in addressing these challenges.
The first part of the presentation will be dedicated to the development
of a priori ROM for linearized FSI problems (i.e. hydroelasticity [3] and aeroelasticity). At hand of a number of examples the computation of
the coupled eigen frequencies of FSI systems by projection on solid dry
eigenmodes will be demonstrated and discussed. The second part concerns
a priori ROM approaches based on the Proper Generalized Decomposition
[4] for nonlinear problems (e.g. geometrically nonlinear elasticity with
follower forces or the steady Navier-Stokes equations). The assumption
of variable separability is promising for multi-parametric analysis and
the methodology will be presented with several uncoupled examples.
Finally, the formulation of velocity-based monolithic fluid-structure
problems will be discussed.
[1] Morand, H.-J. & Ohayon, R. (1995). Fluid Structure Interaction, Wiley.
[2] Ravi, S., & Zilian, A. (2017). Time and frequency domain analysis of
piezoelectric energy harvesters by monolithic finite element modeling.
International Journal for Numerical Methods in Engineering,112(12),
1828–1847.
[3] Hoareau, C., Deü, J.-F. & Ohayon, R. (2019). Prestressed Vibrations
of Partially Filled Tanks
Containing a Free-Surface Fluid: Finite Element and Reduced Order
Models. Proceedings of the VIII
International Conference on Coupled Problems in Science and Engineering,
COUPLED 2019, Barcelona, Spain, June
[4] Chinesta, F., Ladeveze, P., & Cueto, E. (2011). A short review on
model order reduction based on proper generalized decomposition.
Archives of Computational Methods in Engineering, 18(4), 395.
Presentations SS 2019
Program Flyer
"Thermal model order reduction considering heat radiation"
"A priori fluctuation modes for microstructures assembled by means of Wang tiles"
"Data-based surrogate models by kernel methods"
" A semi-incremental scheme for fatigue damage computations"
Presentations WS 2018/2019
Programm Flyer
"Modeling and Control of Tendon-driven Elastic Continuum Mechanisms"
Abstract: In modern robots, joint-mechanisms that are built to interact with the environment
usually features intrinsic passive compliance. Based on this design paradigm, elastic continuum
mechanism are also applied frequently. Actively controlling the pose of the mechanism is
indispensable in robots. However, the soft structure reacts to any kind of external loading or
disturbance. An accurate model that captures all intended deformations is usually computational
expensive and not applicable in real time control. Therefore, this talk will deal with reduced
models for such kind of system that allows for their capability analysis and for model-based control.
"Artificial Neural Network Surrogate Models in Structural Mechanics"
Artificial neural network surrogate models can be applied to several fields in structural engineering, e.g. to replace time consuming finite element simulations for structural optimization, reliability assessment, sensitivity analysis, system and parameter identification, structural health monitoring, real-time simulations for computer aided steering of structural processes, and structural control. This lecture contains an overview on applications of artificial neural networks in structural mechanics. Feedforward and recurrent network architectures and corresponding training algorithms are discussed. Examples for neural network based surrogate modelling of computationally expensive structural models are presented. Also the possibility of neural network based material models within the finite element method is shown. In addition, strategies are discussed to consider uncertainties of structural and material parameters within artificial neural network approaches.
Data-driven microstructure-property relations
Data Driven Parametric Modeling in Discrete Least Squares Norm
Presentations SS 2018
"Space-Time Model Order Reduction for nonlinear path-dependent long-term and cyclic processes"
"Efficient Large Strain Homogenization: Reduced Bases and High-dimensional Interpolation"
"Simulation Data Science – a Case Study on Material Failure"
"Nonlinear model order reduction for explicit dynamics"
"The Reduced Basis Method for Parameter Functions and Application in Quantum Mechanics"
Presentations WS 2017/2018
"Using Feedthrough to avoid unphysical frequencies in reduced systems"
Abstract: Almost all linear model order reduction schemes for mechanical systems achieve static correctness or local precision by adding static mode shapes to the reduction basis. Since this basis is used to project mass and stiffness matrix, these static mode shape develop a entirely unphysical frequency in the reduced system which may cause serious problems if these frequencies are excited. Instead of achieving static correction by using static correction modes, a simple addition to the spectral sum is proposed. This approach has several advantages: The number of degrees of freedom is further reduced, unphysical dynamics are eliminated, the reduction is still statically correct and the numerical efficiency increases considerably. The potential and advantages of the approach will be discussed and demonstrated for numerical test examples.
"Greedy algorithms for optimal measurements selection in state estimation using reduced models"
Abstract: In this talk, we will talk about recent techniques developed to estimate the state of a physical system using sensor measurements and reduced models. After giving a short overview on the methodology and the approximation results, we will explain how we can use the methodology in order to select the sensors to place in the physical system in an optimal way. If time permits, we will also discuss the challenges posed when the the sensor measurements are no longer exact but polluted by noise. This is a work in collaboration with P. Binev, A. Cohen and J. Nichols.
"Automatic derivation of material laws for simulating structural components"
Abstract: In our talk we will present a novel approach to automatically derive material laws by model order reduction methods (MOR) for the component simulation of fiber reinforced plastic (FRP) materials, which is based on the output of
an injection or compression moulding simulation.
"Structure Preserving Model Reduction for Linear Elasticity"
Presentations SS 2017
"Structure-Preserving Model-Reduction"
Abstract: Reduced basis methods are popular for approximately solving large and complex systems of differential equations. However, many challenges remain to secure the flexibility, robustness, and efficiency needed for general large-scale applications, in particular for nonlinear and/or time-dependent problems. In this talk, we present a greedy approach for the construction of a reduced system that preserves the geometric structure of Hamiltonian systems. Preserving the Hamiltonian structure ensures the stability of the reduced system over long-time integration. The performance of the approach is demonstrated for both ODEs and PDEs. We then discuss how the method can be extended to preserve the symmetries and intrinsic structures of dissipative problems through the notion of port-Hamiltonian systems.
"Controlling of the model reduction error in FE2 analysis of transient heat flow"
"Localized Model Order Reduction"
"Variational Inertia Scaling for Explicit Dynamics"
"Kernel Methods for Nonlinear Control and Random Dynamical Systems"
Presentations WS 2016/2017
Milestone-Presentation:
"Error Controlled Nonlinear Model Reduction Techniques for Crash Simulations"
"A Newton-Euler approach to modelling and control of flexible manipulators"
"Homogenization of viscoplastic composites based on the complementary TFA"
14:15, PWR 5a, 0.015
Prof. Sonia Marfia (University of Cassino and Southern Lazio)
"A nonuniform TFA homogenization technique based on piecewise interpolation functions of the inelastic field"
"Kernel Methods for Accelerating Implicit Integrators"
"Reduced Basis Methods for Inverse Problems"
Presentations SS 2016
"Reduced Basis Approximation of the time-discrete Algebraic Riccati Equation"
"Robust optimization of permanent magnet synchronous machines using model order reduction for the efficient computation of local and global sensitivities"
"Efficient finite element simulation for cyclic loads with a viscoelastic-viscoplastic-damage material model"
"Nonlinear modes and their suitability for model order reduction"
"Application of model order reduction techniques to the lubricated contact of elastic bodies"
Previous Presentations
Contact
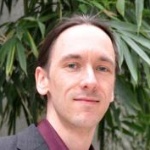