Mathematical models to describe natural phenomena are often based on partial differential equations. The inputs to the equation, such as coefficients, boundary or initial conditions, the data or the domain itself may not be known a-priori. Insufficient or imprecise measurements have to be accounted for when the model is supposed to be realistic. The equation itself could, further, be chaotic. Stochastic partial differential equations account for all sorts of uncertainties and chaotic behaviour. The aim of the group is to investigate solution concepts for stochastic partial differential equations, analyse solutions and invent numerical methods to approximate solutions, hence, bridge the gap between stochastic analysis and numerical simulations. The methods developed have applications in various natural and engineering sciences.
News & Events
-
Modelling Muscles with the Help of HPC
Research area computer-aided modeling of the human musculoskeletal system of Prof. Dominik Göddeke -
HPCwire article
Building resilience and fault tolerance in HPC for numerical weather and climate prediction. -
"Symplectic Model Order Reduction with Non-Orthonormal Bases" published
New Scientific Article
Contact
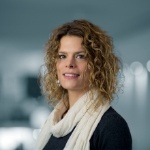
Andrea Barth
Prof. Dr.Head of Examination Committee Bachelor Mathematik B.Sc. Head of Group