To model phenomena in the natural sciences and/or financial markets, oftentimes (partial) differential equations are utilized.The underlying dynamical system may be subject to uncertainty, for instance parameter and domain uncertainty or the driving noise of the system is pertubed by a stochastic process. A common approach to capture this behaviour is to model the source of uncertainty by continuous Gaussian processes, which are analytically tractable and straightforward to simulate. It turns out, however, that Gaussian random objects are unfit to capture the impact of spatial and temporal discontinuities, for example in flows through fractured porous media or composite materials. Furthermore, Gaussian distributions notoriously underappreciate rare events, thus heavy-tailed, discontinuous Lévy-processes are better suited to model stock returns and energy forward markets. We investigate the approximation and simulation of non-Gaussian random objects and the numerical analysis of the corresponding stochastic partial differential equations. To simulate discontinuous Lévy random fields we analyse certain correlation structures and develop algorithms to sample from heavy-tailed distributions. Due to the lack of spatial and temporal regularity, standard numerical methods to solve dynamical systems will be time-consuming or even fail to converge. To this end, we investigate adaptive and stabilized numerical approaches that allow us to estimate arbitrary quantities of interest within reasonable computational time.
For further information please contact Andreas Stein
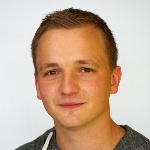
Andreas Stein
Dr.Former Research Assistant