Estimation of model parameters with observed data is an essential part of mathematical modeling and scientific computing. However, parameter estimation poses many fundamental and computational challenges, e.g., ill-posed or non-unique solution as well as highly non-convex optimization problems. Bayesian inversion has emerged as a powerful approach to tackle such challenges, by providing a distribution of solutions instead of a single estimation. Such distribution can then be used to extract the desired information. We aim to apply the Bayesian inversion to partial differential equations that contain random fields with jump discontinuities. Uncertain fields appear frequently in applications, e.g., in porous media fluid dynamics, medical imaging applications and finance, where the correct estimation of parameters is crucial. The Bayesian inversion can significantly improve the estimation of unknown parameters in such applications.
For further information please contact Dr. Babak Maboudi Afkham
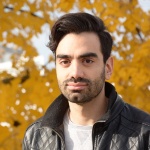
Babak Maboudi Afkham
Dr.Research Assistant